June 10, 2021
AtScale in Action: Scaling Self-Serve BI Program on Snowflake With a Semantic Layer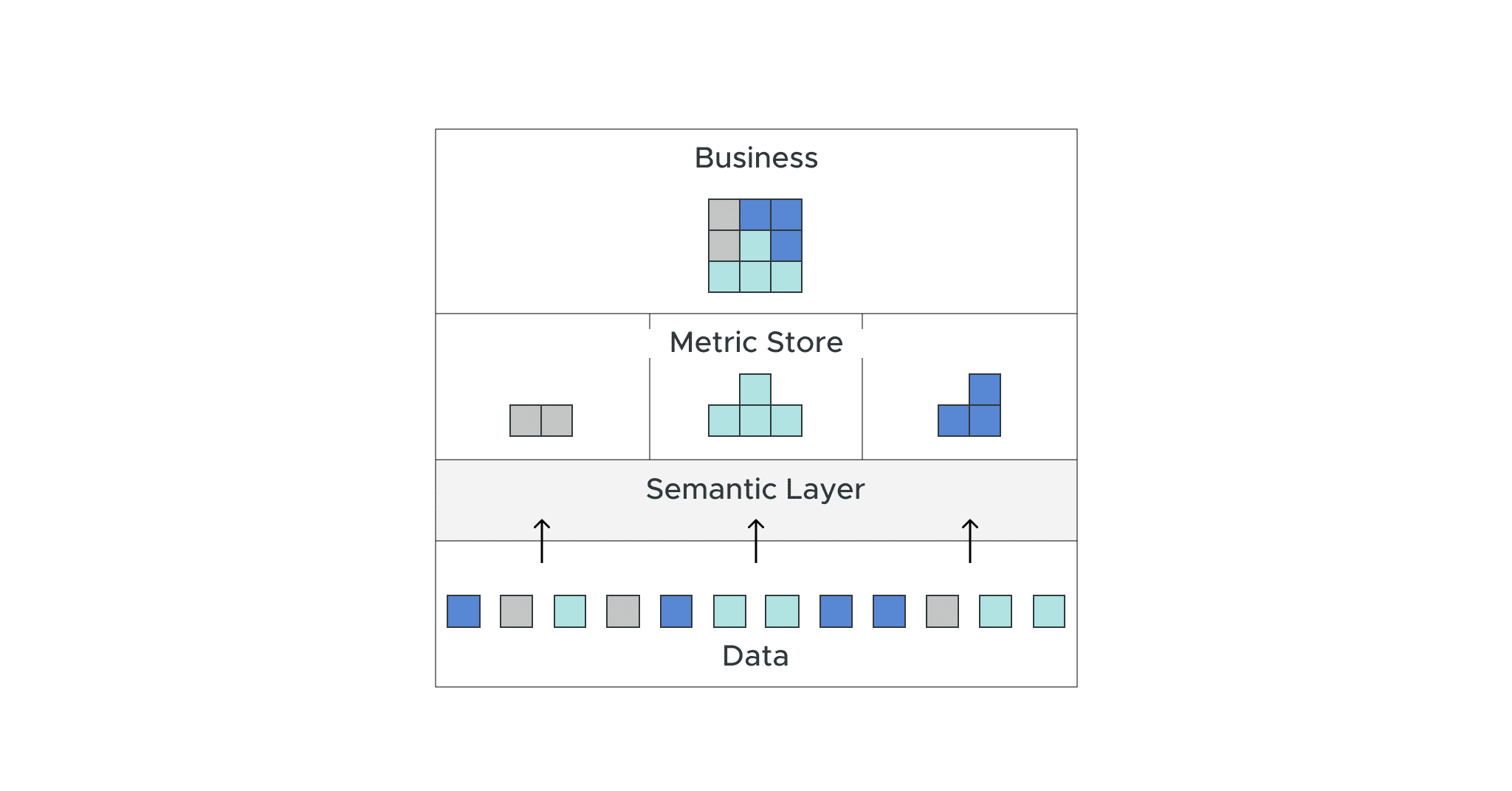
Self-service analytics has long been hailed as a transformative force in data-driven decision-making. However, persistent challenges such as poor data quality, debates over control between IT and business units, and the absence of robust governance controls have hindered its realization. In our persistent pursuit of successful self-service analytics, a perplexing question arises: Why does success seem elusive despite our collective efforts to achieve and enable self-service analytics?
The answer lies in our historical emphasis on addressing the challenges at the interface layer. We’ve witnessed innovations ranging from the drag-and-drop explorative UIs of the early 2000s to rule-based natural language interfaces in the 2010s, and now, the advent of GenAI. However, the crux of the matter isn’t rooted in the interface itself; rather, it resides in the abstraction layer. The core challenge lies in effectively abstracting the business logic that must be consistently applied to the data to ensure its availability across all consumption interfaces. In this blog post, I delve into the heart of this exact problem: the abstraction of business logic and how a metric store serves as a vital bridge between data and business needs.
The Value of a Metric Store
As highlighted in a previous blog post, a metric store acts as a unifying language, providing consistency across consumption interfaces. It addresses the root cause of the challenges faced in self-service analytics, ensuring that business logic is applied consistently to the data. Now, let’s explore how AI can further augment a metric store and propel it into a universal semantic layer, transforming it from a static repository into an intelligent, adaptive system.
AI Augmented Metric Stores:
At AtScale, we envision six core areas where AI augmentation enhances the value of a metric store:
-
- Predictive Analytics – Forecasting: AI analyzes historical metric data to make accurate predictions, enabling the anticipation of trends and proactive decision-making.
- Anomaly Detection – Identifying Outliers: AI algorithms automatically detect anomalies, empowering users to swiftly pinpoint unusual patterns for investigation.
- Personalized Recommendations – User-Specific Insights: AI analyzes user behavior to provide personalized metric recommendations, suggesting relevant KPIs based on individual roles and responsibilities.
- Contextual Insights – Contextual Understanding: AI enhances metric data understanding by analyzing contextual information such as industry trends and market conditions, providing users with a comprehensive view.
- Explainable AI (XAI) – Interpretability: AI models within the metric store are designed with explainability, fostering user trust by helping them understand how insights are generated.
- Automated Alerting – Proactive Notifications: AI-driven alerting systems automatically notify users of significant changes or anomalies in metric data, enabling timely responses to emerging issues.
By harnessing these AI capabilities, a metric store evolves beyond its static nature to become an intelligent, adaptive force. This transformation empowers users with deeper insights, predictive analytics, and a more user-friendly experience. At AtScale, we are enthusiastic about the potential of this evolution, guiding our product direction to lead the way in reshaping the landscape of self-service analytics. Join us on this exciting journey towards a future where AI and metric stores converge to unlock unprecedented value for organizations.
Power BI/Fabric Benchmarks