February 2, 2023
What Is an AI Center Of Excellence?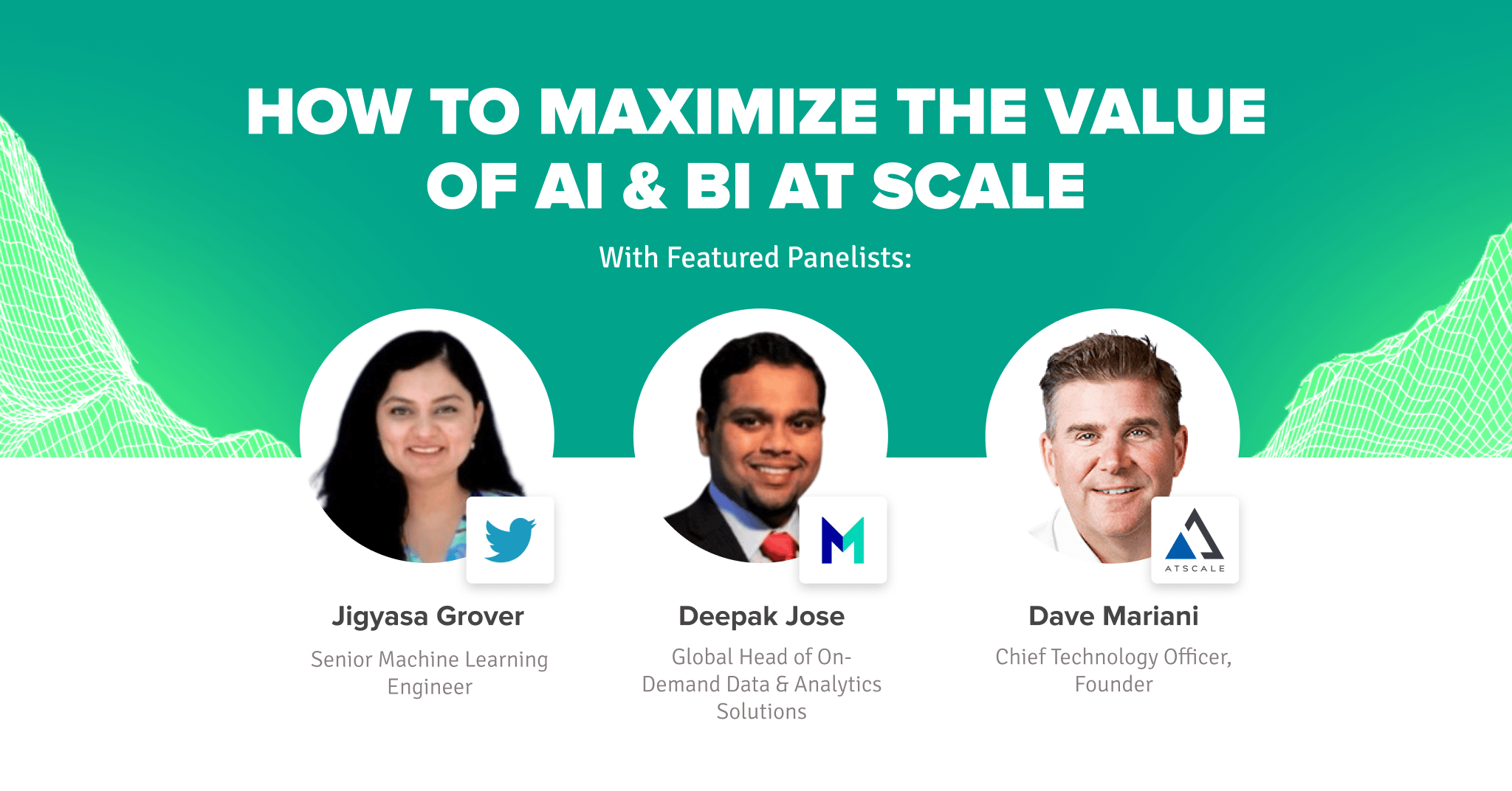
A few weeks ago, I spoke with two industry experts to get a first-hand view of maximizing AI and BI at scale. The panelists included Deepak Jose, Global Head of On-Demand Data and Analytics Solutions at Mars, and Jigyasa Grover, Senior Machine Learning Engineer at Twitter.
The discussion focused on the philosophical and practical aspects of implementing AI and BI in real-world business contexts. Both speakers gave us a snapshot into their brands’ approaches to data and analytics with examples, tips, and insights.
Let’s dive into how Twitter and Mars use AI and BI to create a better customer experience.
Value > Data
According to Deepak Jose of Mars, it’s important to prioritize value when building out AI and BI. Commonly, businesses will pick a solution first, use it to process data and then find business problems that this solution can solve. But Deepak believes that this process needs to be flipped. In his words, “We wanted to start with the value first; that is essentially the decision-back approach. What are the business problems that I can solve?”
Mars’ Customer-First Approach to AI
To prioritize value over data, Deepak’s team uses a customer-first approach rather than just collecting data for data’s sake. He believes all efforts across all business units – including data and analytics – need to prioritize better results for the customer.
According to Deepak, this concept is especially important for companies that set up an integrated consumer journey or omnichannel approach. To truly create a seamless customer experience, your business needs to make interconnected decisions behind the scenes. Otherwise, each business unit will only see its own piece of customer interactions and fail to grasp the big picture. In Deepak’s words, “The sales explain the customer a certain way, the marketing explains the customer a different way, the supply chain explains a whole different way. We are all talking about the same customer. And this is why we need interconnected decisions.”
To accomplish this big-picture alignment from an AI and BI data standpoint, you need to start with the overarching business problems first. Only bring in the solution after you’ve established why that data should be collected in the first place.
Tips for Building out Value-Based AI
Deepak provided a few tips for taking a value-over-data approach to AI, including:
- Ethical and responsible AI. Mars set up parameters such as an AI code of conduct, governance, and sustainability practices to create a responsible data and analytics program. These ethical guidelines strengthen their external brand as a whole, attracting both customers and new talent who care about their brand’s meaningful and proactive approach to social responsibility.
- Cultural and change management. Any plans to introduce new technology need to be actionable. Your data analytics team might think that a change to your AI approach is a great plan, but the rest of the company might see it as an obstacle. So, you need to give everyone a clear direction forward. To do this, plan for upskilling, training, and handling organizational culture shifts.
- Small, but courageous next steps. In Deepak’s words, “The biggest thing that holds us back is the status quo. You need to have the courage to challenge the status quo to make a significant change.” Change is not easy, especially for large companies with lots of moving parts. So to move growth in a positive direction, stand up for your ideas with courage. But, be sure to start small.
AI and BI at Scale, a Real-World Example
During the second half of the webinar, Jigyasa Grover of Twitter presented a practical example of predictive modeling at scale. Her team’s approach to scaling AI and BI is all about balancing computational and business goals.
Twitter’s Personalization Efforts
Today, all social media platforms face a common challenge: adapting to a user base that wants to see high levels of personalization without compromising other areas of the user experience. One of Twitter’s personalization efforts ranks conversations based on user preferences. It does this by prioritizing which comments are seen first every time a user clicks on a conversation.
How Twitter Prunes low-Quality Comments with ML
While these personalization efforts are important to the user experience, they also take up a lot of computing power. To solve this issue, Twitter uses an ML model to prune low-quality comments. They aim to decrease the system load without compromising business insights or customer experience. Here’s how Jigyasa walks this balance between business and computative goals:
- Measurement. Jigyasa’s team collects metrics that indicate their system’s health and identify areas of improvement. A few examples of these metrics included success rate, throughput, and latency.
- Quality. The Twitter team needed an ML model that could quickly identify the quality of each comment. So, they needed to decide how to collect these signals. Jigyasa recommends using your business goals to decide whether model-based or rule-based computation is a better choice.
- Quantity. Jigyasa’s team also chose a system load factor to determine how many comments would get displayed to each user. But, they also had to consider if this factor would affect the user experience under various circumstances (i.e. system is not under load, there are only a few conversation participants, etc.).
- Experimentation. After working with those first three factors, the Twitter team used A/B testing to experiment with their new ML. Jigyasa emphasized that their business goals had to stay front-and-center, throughout this experimentation process. Regardless of changes made to the machine learning model running behind the scenes, “user experience should not be affected, or any of the other metrics. The click-through rate, or revenue, or any other thing that you use to measure your success should not get impacted.”
How a Semantic Layer Brings Value to AI and BI
A “value first, data second” approach requires all hands on deck to succeed. Data analytics don’t just exist in a vacuum. Instead, they affect all other areas of business: marketing, sales, customer success, etc. Because of this, your team’s AI/ML models need to match the big-picture goals of the entire business.
As we heard from Twitter and Mars, maximizing value at scale takes company-wide buy-in, good cross-team communication, and a deep understanding of your customers’ needs. Here at AtScale, we help teams accomplish this by adding a semantic layer to their AI and BI data. We define a “common language” for your data to make it more accessible and cohesive to your entire organization. An independent semantic layer leads to better data literacy and more self-service modeling and analytics options. Plus, it makes your data scalable and flexible to the platforms that your teams use on a daily basis, fostering a collaborative approach to data and analytics.
AtScale is here to help you go beyond “data for data’s sake” and deliver higher customer value with your AI and BI. To learn more, listen to my full conversation with Deepak and Jigyasa in the webinar, “How to Maximize the Value of AI & BI at Scale”.
RESEARCH REPORT