November 1, 2021
Why You Need Next-Gen OLAP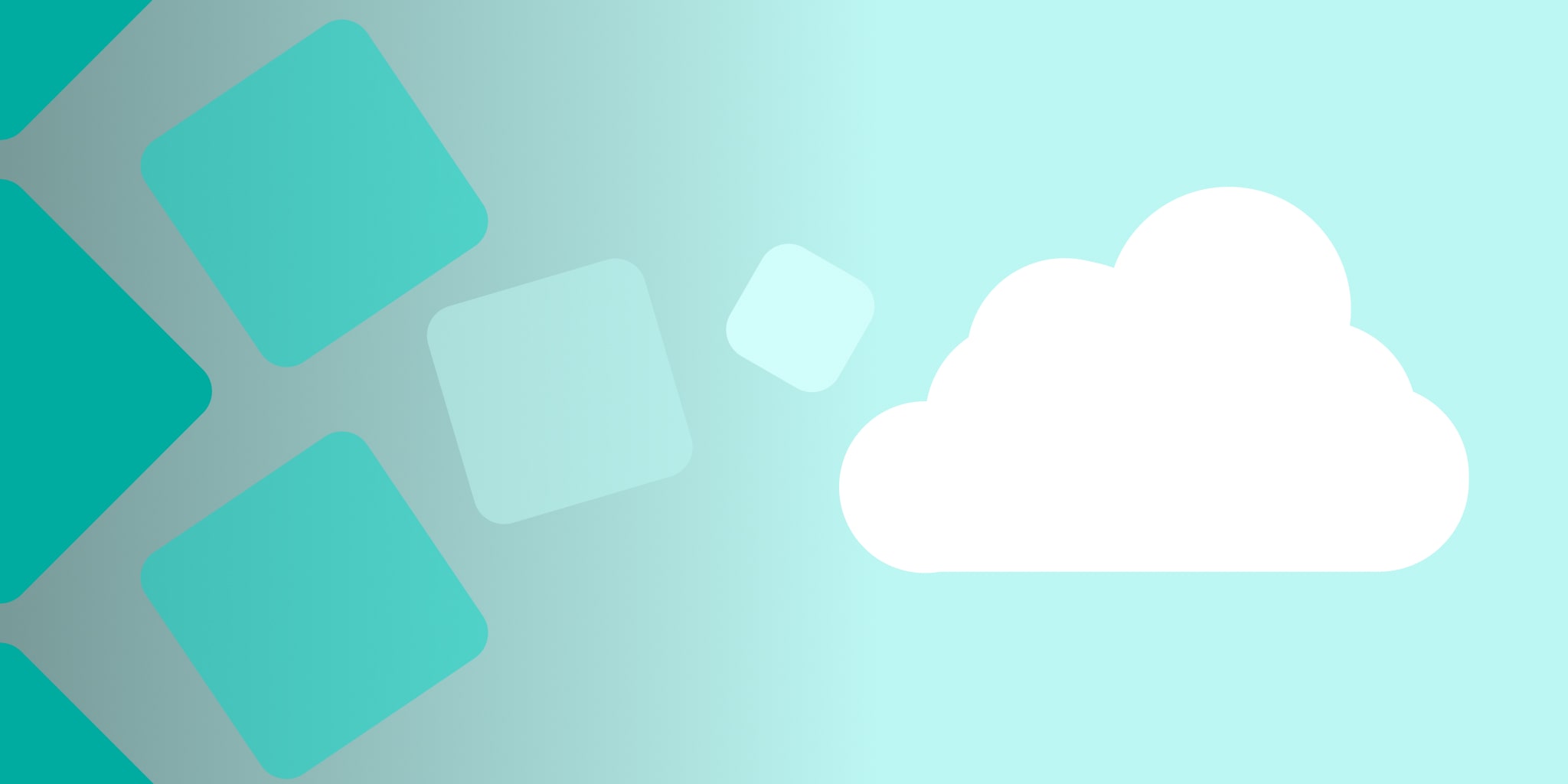
Where is the industry going in terms of large scale data and machine learning data? We’ve made fantastic strides over the last five-eight years, but business-centric analytics has not reduced in value.
Much of the business intelligence and data science companies focus on something a little bit different and in doing so, what AtScale has said is, “Hey, let’s not forget about the business-centric analytics. How do we bring multiple data sets together, handle large scale data, incorporate machine learning while delivering it in business context? ”
Widespread Support
In retail (or any organization really), you have things like a warehouse and inventory control. You want to combine that with your sales metrics, and you want to combine that with other types of third-party data. That kind of business analytics have largely been done through BI tools like Excel, Tableau and Power BI.
We have to be able to support the business analytics by supporting the tools they use really well. BI tools speak different languages. Without getting too technical, Excel speaks MDX and not SQL. MDX is a robust language designed to both express a query, but also displays the business construct of the data. It’s not enough to only be able to commit and answer the query, but it’s also about how we present the data to the end user. Supporting Excel means supporting pivot tables, so the data is represented in a way that the business understands it and can ask smart, intelligent questions of the data.
With Tableau, AtScale exports a TDS (a Tableau data source). And in that data source, we effectively present the data with hierarchies, folders, drill down paths, etc. With this, the business knows how to effectively use that data. You don’t have to learn it or do your own calculations. It has already been done. Now you can just use the data with governance and confidence. And the same thing is true for Power BI.
Power BI as well, has a way to present the data. The same business semantic definition of the data is found in all BI tools. PowerBI’s MDX connector to AtScale preserves the OLAP concepts baked into the virtual cube. These are the things that are important for the consumption of data by people who are not necessarily super technical in the weeds of what the data structures look like. For Power BI, we’re expanding our relationship with Microsoft. There’s more to be said here in the coming months.
Understanding the Data …
As an industry, we’ve gotten to the point where we’re just trying to present big, fat, and wide tables; expecting the business to know what we’re talking about. The net positive thing is we’ve got so much data. But the negative is that again, the business needs to be able to understand it.
From an AtScale perspective, we talk about the Universal Semantic Layer™ and that semantic layer is the ability to get business context out of this data. When you have a measure available, like available beds in hotel rooms versus inventory versus profits and revenue; the way we calculate those things sound very simple. But in fact, it can be very complex.
When you have multiple streams of data, oftentimes the business has to check multiple streams of data to get the right answer. When you encapsulate that inside of the AtScale model, there’s a lot of governance and a lot of confidence that comes with that because the business person who’s now asking the question doesn’t have to redefine what that metric means or how it’s derived.
The other thing that’s really important is AtScale’s ability to support complicated business logic in metrics. When we think about OLAP functionality and business analytics, there’s a lot of techniques that the business uses to actually come up with a calculation and they tend to be very different depending on the level of the hierarchy that you’re looking at. For example, how you measure visits to a store or how you measure inventory, at a grand total can be very different than how you measure it, at an individual warehouse level or individual state level. So we have to be able to redefine that measure based on the level of the hierarchy. And these are things that AtScale allows you to do.
When we think about presenting the semantic layer, the definition of the data, the business can intuitively understand what that data is. Ultimately the goal is to have the confidence that regardless of what tool they might be using, if it’s Tableau or Excel or something else, AtScale is going to return the same answer and that the calculation is consistent across whatever they do.
OLAP Isn’t Dead
OLAP is an old term. We think about it in terms of financial reporting. But OLAP is effectively multi-dimensional analytics. I’ve seen this everywhere. What is dead is pre-baking an entire OLAP cube by default.
For example, if you want to understand network behavior, you would take a look at web server data that’s internal and look at what employees do when they’re at work (what sites they’re going to internal and external). This type of cyber analytics includes streams of data that is very granular like network traffic, but it needs to be combined with data from HR for a deeper understanding. HR data comes at the employee and day level. Making sense of these disparate data sets with their own set of attribution and recorded at much different levels of granularity is the complexity, but offering the business analyst (or the cyber analyst) an easy to understand data service is the key for adoption.
Making Logical Decisions with OLAP
OLAP can be used in multitude of ways. You can have inventory analysis where OLAP has been very traditionally used. Inventory analysis is important because you want to understand the first and last child type of measures. For example, if you make Teddy bears and you have 10 teddy bears in your warehouse at the end of the month, you don’t have 300 after 10 days. You want to be able to check first or last day to be able to figure that stuff out. If you’re doing sales and forecasting analytics, you want to deliver the analyst the actuals when they exist and the forecast when actuals don’t exist. Of course Forecasts are done at the regional level, and actuals come in at the store level. And all of this stuff is all OLAP analytics. There’s a lot of technical underpinnings to that, but when the end user uses that data, they shouldn’t need to know all of the technical underpinnings. You just have to make logical business sense. It’s not always just, “I want to sum every row”. That’s a very simple thing to do, but the constructs of the business to understand hierarchies, to understand unrelated dimensions, this is what we’re talking about with OLAP, and it’s really cross functional and across multiple types of industries.
And What About Compliant Data?
That definition of data (that semantic layer in AtScale) is built once, and multiple tools will then access it. What we don’t want to do is redefine this across the different types of BI tools. AtScale can do this while providing speed of thought query time with our Autonomous Data Engineering.
Effectively we are a virtual cube. But in order to build that speed of thought performance, we begin to learn from our analysts’ and developers’ behavior, the inbound query and the query patterns. AtScale is using all that information to autonomously build an optimization layer we call Acceleration Structure.
That optimization layer means that as different people begin to log on to their BI tools and ultimately query through AtScale, AtScale is predicting those types of questions. It’s storing answers to questions we believe you will ask based on your other inquiries. So that means that you will have the consistency of definition, but you’ll also get a very quick type of response time. And those things together builds confidence in the system. It’s an important thing to be able to provide data that the user can understand that doesn’t take half an hour to get an answer from when you query it.
ANALYST REPORT