June 16, 2021
How Insurance Companies Can Merge Foursquare Places Data with First-Party Data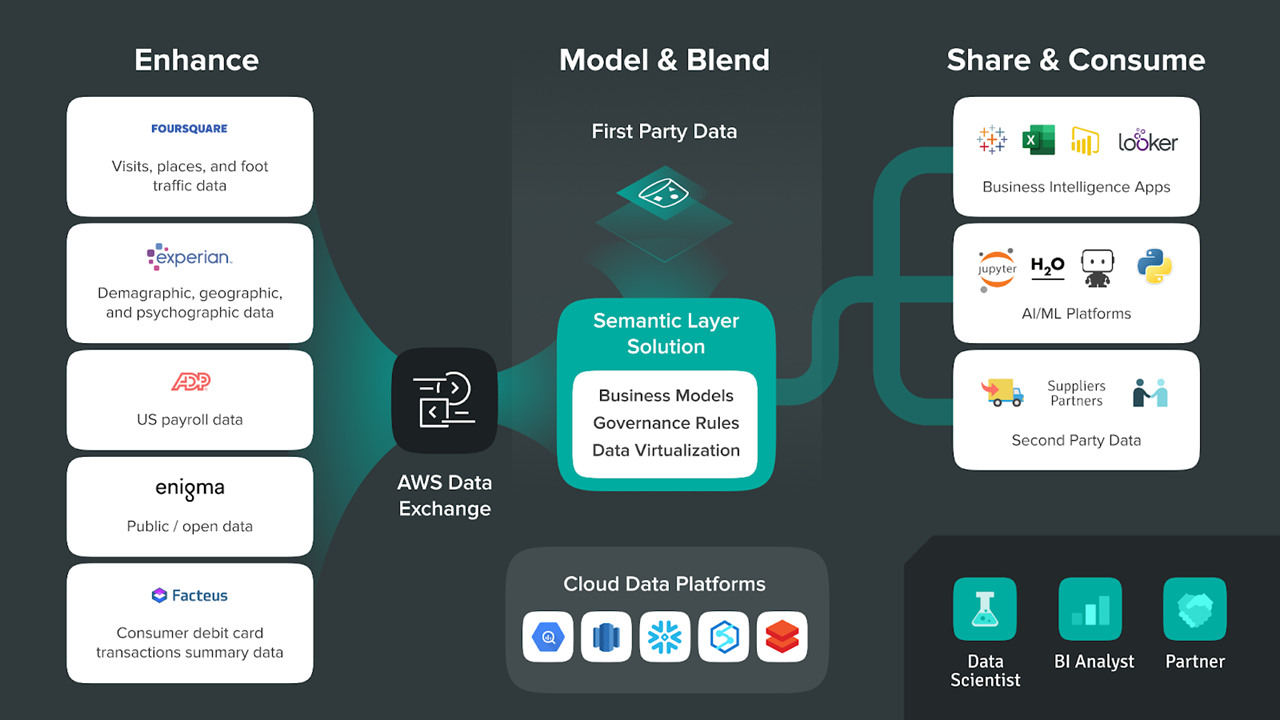
While the use of external data has been around for a long time in some industries, the demand for more data sources continues to grow across all verticals. In fact, research by Forrester and Pitney Bowes found that 92% of analysts believe their organization needs to increase the use of third-party data. That’s because enterprises that manage to blend data from multiple sources have the opportunity to leverage far more information to perform advanced analytics and machine learning.
As more companies recognize the potential competitive advantages of external data, the need for a streamlined architecture to better operationalize data will increase. In this post, we’ll take a look at the benefits of third-party data and how a semantic layer can facilitate data sharing.
What is Third-Party Data?
Third-party data sharing is the exchange of data with external stakeholders and other public data sources. This differs from first-party data sharing internally within your organization and second-party data sharing with your vendors or business partners.
The challenge with the external data ecosystem is that it is fragmented across a diverse set of data brokers, data aggregators, and other types of data products. That’s why many organizations use a solution like AWS Data Exchange, which has consolidated the data from hundreds third-party providers into a centralized service. These data exchange services make it easier for companies to subscribe to external data sources, but as we’ll discuss later, there still needs to be a way to combine this third-party data with existing data.
The Benefits of External Data Sharing
Although many organizations already use internal data for analytics, external data can further improve decision-making based on advanced analytics and predictive algorithms. Here are a few reasons why.
For one, external data can enhance internal datasets by adding context and closing any knowledge gaps. Organizations may generate a lot of data from day-to-day operations, but this limits the quality of insights advanced analytics can deliver. Using external data, business analysts can get a more complete picture of how the enterprise fits in the broader business environment to drive decisions-making.
For example, there are a number of external events that can impact an organization – from consumer trends and competitor initiatives to changes in the economy. More comprehensive datasets that include internal and external sources can help businesses account for these potential opportunities and risks during the decision-making process.
Further Reading: Using Data Sharing to Make Smarter Decisions At Scale
Blending datasets also can also create better outcomes from predictive analytics models. That’s because unifying different data sources that would not be combined otherwise can allow machine learning algorithms to perform a deeper analysis. There’s a limit to how much useful data an organization can generate on its own, but supplementing internal data with external sources can create larger and more rich datasets that may improve the results from machine learning algorithms.
How a Semantic Layer Facilitates Data Sharing
As more data moves into the cloud, it’s becoming easier than ever to perform advanced analytics, machine learning, and more. While the cloud has made it cheaper and faster for many organizations to work with first-party and second-party data, there’s been a lag in adoption for third-party data.
The main challenge with third-party data is that it’s difficult to use with existing data sources, yet blended datasets are where the greatest insights are often found. AtScale’s semantic layer uses live connections with on-premise and cloud data sources to break down internal data silos and make external data more accessible.
In addition, data virtualization enables companies to share third-party data throughout the organization without expensive and time-consuming data movement and transformations. Data virtualization hides the complexity of dealing with multiple data sources, so that business analysts and Data Scientists can more easily consume blended datasets within popular BI (e.g. Power BI, Tableau, Excel) and AI/ML tools (DataRobot, H2O, Python)..
As the amount of data being created and shared continues to accelerate, it makes sense for enterprises to tap into these big data sources in real-time to make smarter decisions. Using AtScale, organizations can enable self-service analytics with both internal and external data at scale. This allows companies to enhance their existing datasets to achieve greater growth, productivity, and other positive business outcomes.
Want to learn more about third-party data sharing? Listen to our webinar with Foursquare and AWS: “How to Merge Places (POI) Data with Additional Datasets to Make Smarter Decisions.”
ANALYST REPORT