November 29, 2021
How to Build a Feature Store with AtScale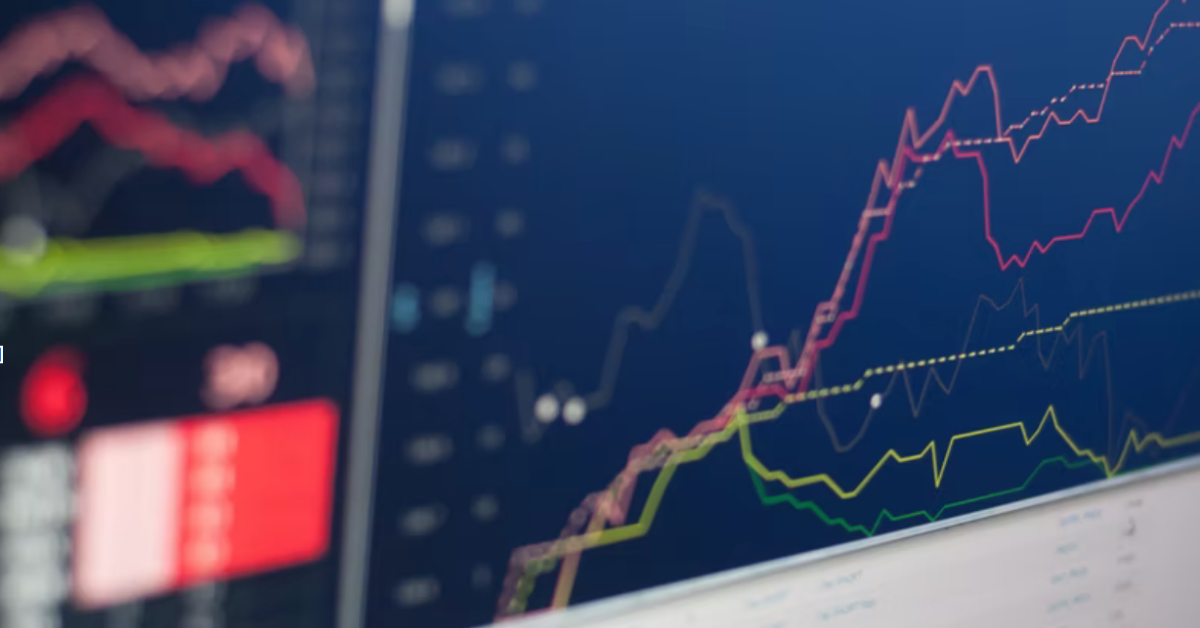
AtScale has been helping bridge Enterprise BI and Data Science for years, recently announcing AtScale AI-Link to simplify access to our semantic layer platform with a Python library designed for data scientists. We clearly see an explosion of interest around data science and enterprise AI and often get involved in conversations on how AtScale can help.
We consistently hear three basic challenges to scaling enterprise data science programs. First, there is a clear shortage of data science skills relative to demand that will be the case for years to come. Second, keeping data scientists productive is complicated by the amount of time they typically spend wrangling data (vs. applying their knowledge of sophisticated model development). Surveys of data scientists show 40-60% of time is spent working with raw data. Third, organizations are challenged to demonstrate return on data science investments. Data science programs deliver hard value when AI/ML-generated insights get in front of decision makers at the time of decision. These challenges are most significant as organizations scale.
AtScale’s semantic layer platform can be a key enabler by addressing some of these fundamental challenges head on. Here are 10 important ways an AtScale semantic layer can help.
1. Building off existing BI team investments
The concept of a semantic layer has been part of business intelligence strategy for years. Even if there is no formal semantic layer solution, BI teams will have put thought into creating a single source of enterprise metrics and analysis dimensions that different consumers can draw from. AtScale builds on this concept with an independent semantic layer platform that abstracts the complexity of raw data from consumers and ensures high performance live query access to underlying cloud data data platforms. As underlying raw data changes, a semantic layer can insulate data consumers, including data scientists, from disruption by maintaining a consistent set of enterprise KPIs.
Production ML models can draw on this controlled set of features to ensure consistency and availability.
2. Ease of integration with AutoML and Python Notebooks
AtScale AI-Link provides a basic python library to inventory available features and pull data from live cloud data platforms. As a python call generated from an AutoML platform (e.g. DataRobot) or notebook requests data, AtScale generates a real-time SQL statement optimized for the underlying cloud data store (e.g. Snowflake) and returns results via Python. This eliminates the need for data scientists to navigate the details of querying the cloud data store directly.
3. Curated, Governed Metrics and Dimensions
AtScale provides a layer to curate analytics and apply governance policies. As we have written before, governance is key to self service as it gives data consumers the confidence they are using the the right data to calculate key metrics (e.g. revenue, shipments, headcount, etc…) and using the right analysis dimensions and hierarchies (e.g. time, geography, product family). This confidence helps data scientists work faster, with no need to check and double check they are operationalizing models based on the right features. Further, as underlying data structures change (as they always do), data ops teams can insulate existing analytics and models from this change. AtScale has supported organizations shifting their entire data infrastructure from on prem to cloud, or from one cloud to another, with no disruption to production models.
4. Calculated Metrics
Beyond basic metrics, the AtScale semantic layer enables teams to maintain consistent definitions of more complex, calculated metrics. For example, gross margins, average selling price, cost per user, revenue per employee, or elasticity metrics can be defined and maintained within AtScale. This ensures consistency across different models and teams, radically simplifying the queries to access this data. Data scientists can pull the calculated metric, at any aggregation, and let AtScale manage the query of the source data.
5. Time-Relative Metrics
Building on the point above, AtScale makes working with time-relative metrics (i.e. period-over-period change) simple. As the cornerstone of time-series prediction models, features based on time-relative metrics are fundamental to most every data science program. AtScale simplifies the creation of any number of lag or window based metrics. Further, it is simple to use a customer definition of time (e.g. fiscal periods, work weeks) in the calculation of time-relative metrics. As mentioned previously, the key benefits here are consistency, simplicity, and resiliency
With AtScale modeling canvas, you can define time-relative comparisons of unit sales. Once defined, these metrics will be automatically maintained as raw data refreshes.
6. Blending Data Sets
AtScale provides a modeling environment that lets data teams blend disparate data sets to create a richer set of features on which to build models. We have discussed the ease of combining data from different enterprise data sources (e.g. CRM and ERP systems) as well as the potential of incorporating 3rd party data sources (e.g. from Amazon Data Exchange or Snowflake Data Marketplace). Models that blend data expose uniform dimensions (e.g time, geography) that can be used to build an expanded set of features for models.
7. Accelerate Feature Engineering
The combination of calculated metrics with the ability to incorporate new data sources and aligning key dimensions simplifies feature engineering. Data scientists can experiment with a broader set of features in models and ensure they can ensure feature consistency as models move into production. Further, as an extension of points 4 and 5 above, AtScale can simplify the creation and maintenance of time-based features that are the basis for time-series prediction models.
8. Simplifying Data Pipelines
No matter how complicated the set of metrics or the number of blended data sources, the AtScale semantic layer insulates data scientists and AutoML platforms from needing to build or manage a data pipeline. AtScale manages query translation and query performance with no ETL of raw data.
9. Publish Model Results
AtScale AI-Link is a bi-directional Python-based connection to the semantic layer. In addition to pulling data out of cloud data stores to fuel ML models, it is also possible to publish model outputs (i.e. predictions) through the semantic layer. This enables data teams to more broadly publish insights using existing business processes and BI platforms. For instance, an executive could see forecasted sales in the same dashboard as historical sales. The ability to reach broader audiences is key to realizing the value of data science investments.
10. Results Exploration
Building on the last point, publishing modeled insights within a semantic layer based on a dimensional model brings deeper business context to understanding predictions. The ability for a decision maker to “drill down” on a high level forecast is more impactful than a static report. For instance, the manager of New England sales can look at a regional sales forecast and choose to drill down to state level or store level sales. AtScale lets data science teams leverage existing BI reporting infrastructure to publish model results in existing dimensional analysis tools.
Summary
This blog has summarized how AtScale can help teams architect a highly scalable data science program that gets more from existing data infrastructure. We see AtScale customers making their data scientists more efficient, spending less time on data wrangling. They are more productive as they consider a broader set of features in their models. They establish more robust and resilient data pipelines that ensure that models stay operational even as underlying data shifts. Finally they deliver faster time to value for their organizations as they expand visibility to model outputs and integrate model-generated insights into existing business processes.
RESEARCH REPORT