February 1, 2024
From Data Products to Analytics Products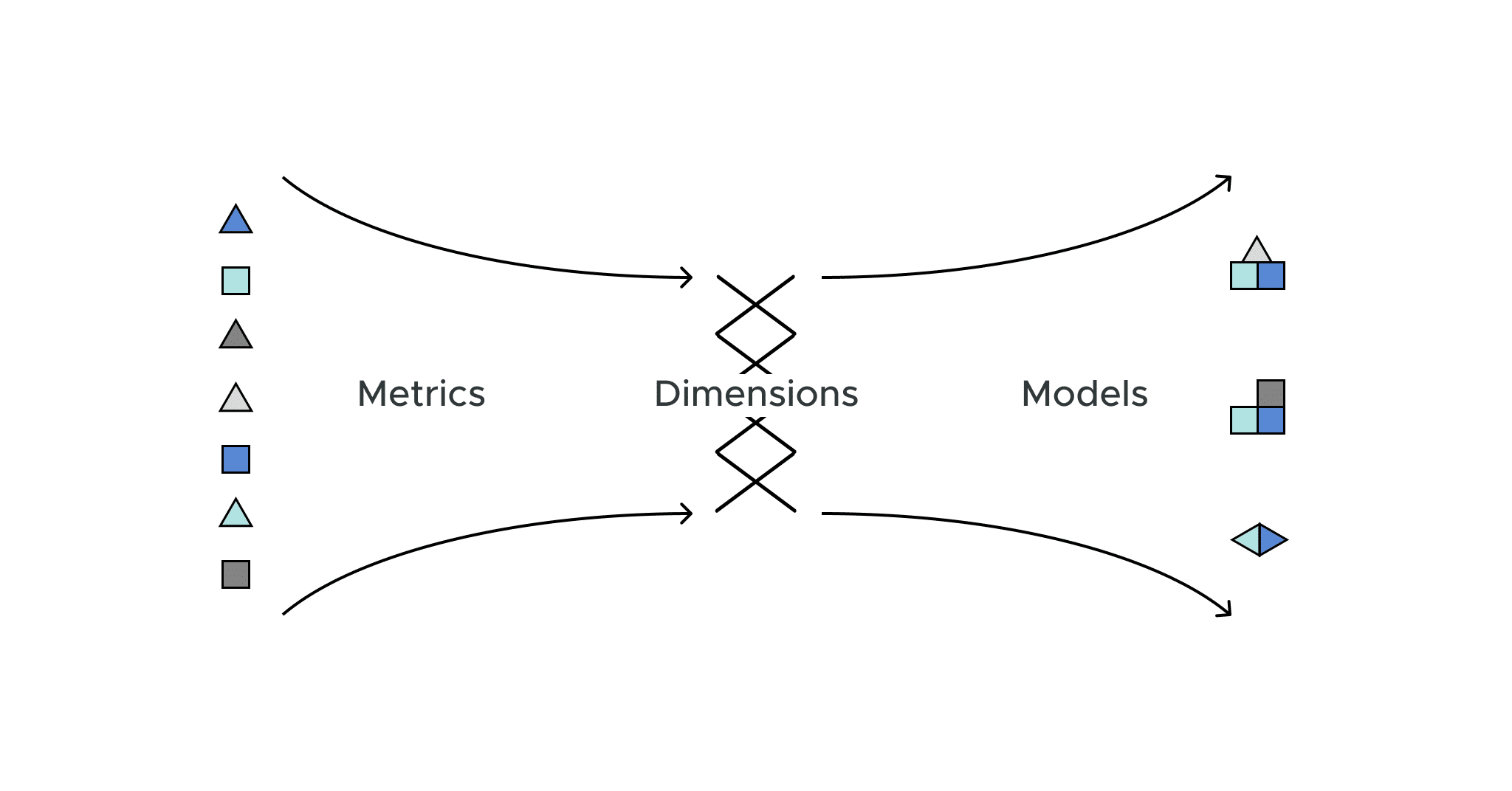
As we covered in parts one and two of this blog series, organizations establish data mesh in order to create more widespread, flexible data access across the entire business. And in order to achieve this, a data mesh approach must enable domain experts to build their own data products. This idea of self-service data products is fundamental to data mesh.
The best way to achieve this is by using “composable analytics” — a library of centrally-governed building blocks for composing new data products. This standard library of assets needs to be created with both shareability and reusability in mind. In today’s blog post, we’ll be covering why this process matters to a data mesh approach and how to accomplish it with a universal semantic layer.
How Composable Analytics Relate to Data Mesh
Data mesh, as we discussed in part one of our blog series, focuses on building an analytics architecture in which business domains take responsibility for their data. But, how do you hand different business units, who each have a varied level of technical and analytical expertise, the right tools for building their own data products? And how do you accomplish this approach without creating discrepancies between different siloed domains?
Gartner notes this dilemma:
“The rise of business technologists means greater demand for self-service capabilities and faster delivery of analytics solutions. Fixation on self-service has created governance issues, such as a change of roles and processes toward greater collaboration between decentralized data and analytics (D&A) and IT. Siloed self-service analytics fail to effectively reuse the value created within accumulated information assets. It is unfortunately typical that many similar analytics outputs have to be built from scratch, wasting a lot of “reinventing-the-wheel” efforts.”
The solution? Gartner recommends modular, analytics building blocks — also known as “composable analytics.” It’s all about establishing standard processes, and, in Gartner’s words, “incubating popular and reusable steps in the self-service analytics process and registering them in an analytics catalog for future composition.”
But all of this can be challenging to achieve. It takes organizational-wide standardization to make these “building blocks” interoperable and flexible enough. And they have to be versatile — able to be shared and reused across siloed departments.
The Common Building Blocks of a Composable Analytics Strategy
In our first two blogs, we covered AtScale’s perspective of data mesh. We mentioned how a semantic layer enables the creation of business-ready data, creating a balance between centralized governance and flexible, independent data usage through a “common language.”
It turns out that a semantic layer can solve this other data mesh challenge: the challenge of creating composable analytics that enables organizations to create and share common “building blocks.” A semantic layer enables the creation, maintenance, and governance of three shareable and reusable components: Metrics, Conformed Dimensions, and Models. These three objects serve as the base “building blocks” for all teams to use, leading to a true data mesh approach. Here’s how work groups use each of these objects to compose new data products within their domain-specific perspectives:
1. Pre-built, Reusable, Governed Metrics
A semantic layer enables consistent versions of primary business KPIs, such as ARR, ship quantity, etc. This nomenclature eliminates the chance of different groups presenting different versions of the truth. It also provides business-vetted calculated metrics, created by the right subject matter experts within the organization. An example could be gross margin, as defined by finance. Once defined, these metrics are searchable within the semantic layer, able to be consumed and implicitly trusted by others. A semantic layer enables organizations to create time-relative metrics as well, eliminating the need to rebuild complex calculations such as quarter-on-quarter growth.
2. Pre-built, Reusable, Conformed Dimensions
A conformed dimension has the same meaning across various data contexts – bringing standardization to important dimensions common to multiple data formats (i.e. from different application sources). They allow measures to be categorized and described in the same way across multiple facts — a “master dimension”, in other words. Their content has been agreed upon across the organization so they can be used to prove business-wide compliance. A few examples of conformed dimensions include time, product, geography, and customer. These conformed dimensions allow reusable aggregation paths for measures across multiple fact tables.
Conformed dimensions are ultimately the “connective tissue” that enables data modelers to blend disparate data sets. This means that everyone in the organization has the same way to drill down in the data and with a single version of truth. As an example, a business that’s analyzing time-based outputs could drill down from annual-level to quarterly-level data, or drill up from quarterly to annually, or anything in between. Dimensions provide the flexibility that teams need in order to connect data sets and contextualize data and analysis.
3. Pre-built, Reusable Models
A semantic model is a logical definition of a new view of data, based on data assets within a centralized data platform. Models enable businesses to further define analytics-ready views of raw data assets, simplifying data interaction for data consumers.
This analytics-ready data can simplify highly normalized data sets. It can also create a view of blended data assets, such as a composite of CRM and finance data or a view of marketing and economic data sourced by a third party. A reusable reference model, defining how two or more data sets get blended, establishes a formulaic approach to blending data sets. It can then be used across multiple end data products, saving time and effort on a normally-complex process. An intelligent semantic layer can help with models as well. As users compose different objects (measures, dimensions) to create a model, the semantic layer automatically provides suggestions on how to connect these objects to create the full data model.
Shareability and Reusability: Key Tenets of Data Mesh
A data mesh approach is all about shareability and reusability. This is why composable analytics is an important aspect of setting up a data mesh within your organization. It encourages the sharing and reuse of analytics building blocks across work groups. Composable analytics also enable a hub-and-spoke model: striking a balance between centralized governance and independent data product creation for each business unit.
To create standard governance guardrails, centralized teams maintain fundamental building blocks, such as conformed dimensions and basic views of analysis-ready data. But at the same time, work groups are allowed to build and govern domain-specific elements of models, minimizing the amount of duplicative work and the risk of mistakes. And by taking a data mesh approach with composable analytics, your business analysts get to spend more of their time analyzing data, rather than waiting on data specialists to create a dimensional data model. With composable analytics, they can easily find the components to build a business ready view of the data, then start analyzing it right away with trust.
Semantic Layer Platform
A semantic layer platform like AtScale snaps seamlessly into your data tech stack, transforming all types of data into standardized, composable analytics. We enable organizations to manage shareable elements centrally while simultaneously empowering data modelers embedded with work groups to create new data products independently.
Learn more about how the semantic layer creates a “common language” for data, making it simple to turn your data into composable analytics and facilitate an organization-wide data mesh approach, or read the entire data mesh series in this white paper, “The Principles of Data Mesh and How a Semantic Layer Brings Data Mesh to Life“.
ANALYST REPORT