February 8, 2022
Developing Smarter, Faster Insights from Business Intelligence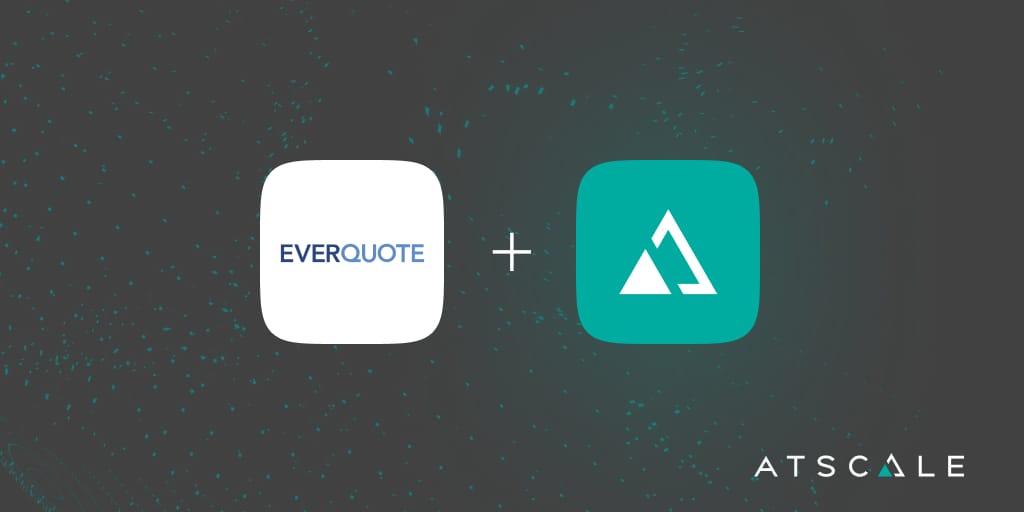
During our recent webinar on scaling self-service analytics, AtScale spoke with Kwan Lee, EVP of Engineering at EverQuote about its multifaceted self-service approach to data analytics for business users and machine learning.
EverQuote operates a leading online insurance marketplace, connecting consumers with insurance providers. The company’s mission is to empower insurance shoppers to better protect life’s most important assets—their family, property, and future. The Company’s vision is to become the largest online source of insurance policies by using data and technology to make insurance simpler, more affordable and personalized, ultimately reducing cost and risk.
Here’s a quick overview of EverQuote’s migration to self-service data analytics.
Data-Driven From the Beginning
EverQuote was formed in 2011 as a spinoff from Cogo Labs, a technology-driven venture accelerator. Since Cogo Labs had a data warehouse for analyzing real-time data about different internet marketing channels, the company was able to recognize an opportunity within the insurance vertical.
As a data-driven company from the start, EverQuote leveraged the technology from Cogo Labs to build a large user base in the insurance space. The original data architecture at EverQuote was a MySQL cluster, various Python services, direct connections to Excel, and a custom online analytical processing (OLAP) interface with point-and-click tools. These roots in data and analytics from the start put EverQuote ahead of the competition when it came to adopting self-service analytics.
“From the very beginning, the company was started by engineers and analysts, which made it a little bit easier to adopt self-service analytics,” Lee explained. “But everybody from business people to customer support still needed to be able to utilize these tools.”
The Need For Scalability & Self-Service Data Analytics
While EverQuote was able to grow its business with its existing data architecture, the company realized that these custom solutions weren’t scalable. Its existing workflow was slowing data analysts down, limiting the company’s potential use of machine learning, and failing to democratize data throughout the entire organization.
The company had decided to shift its ETL process to open source tools like DBT and Apache Airflow to schedule and perform data transformation tasks. Meanwhile, EverQuote used Databricks for several years, but getting data ready for analysts could still take hours. The company needed to streamline its data architecture to make data immediately accessible and easy for anyone to use, not just the data analytics team.
“As the company grew to a greater number of people, it became evident that some of our custom-engineered tools weren’t feasible to maintain long-term,” Lee said. “We also realized that with our existing architecture getting data ready for analysts took much longer than we wanted.”
A Modern Data Architecture With AtScale
EverQuote recognized that the company had grown beyond a team of just engineers and analysts, so it was now long easy for many employees to use data as a strategic asset. The company formed a data analytics team that began promoting data literacy by training and supporting business users in data analytics best practices.
For easier access to data and real-time analytics throughout the organization, EverQuote also decided to adopt Snowflake. In order to make self-service possible, however, the company needed to shift away from its custom OLAP interface to a solution that could make Snowflake the source of all its data.
“We found AtScale and it became the right tool at the right time to revamp our custom OLAP tool,” Lee explained. “In 2020, we adopted Tableau, and this year we started allowing business users to create data cubes in AtScale, which has been very useful for our organization.”
By adopting AtScale’s semantic layer, EverQuote has made self-service analytics at scale a reality. In fact, the company now has over 85 databases and 1500 tables on Snowflake that are now accessible by business users in Tableau and Excel, connected to machine learning workflows, and leveraged for many other use cases. While it took up to a month to make new data accessible to analysts in the past, EverQuote expects to bring this down to just a couple days with AtScale.
Want to learn more about scaling self-service data analytics with AtScale’s semantic layer? Watch our full webinar with General Mills, EverQuote, and Affinity Federal Credit Union here.
Power BI/Fabric Benchmarks