October 18, 2019
Should You Build or Buy Your Universal Semantic Layer?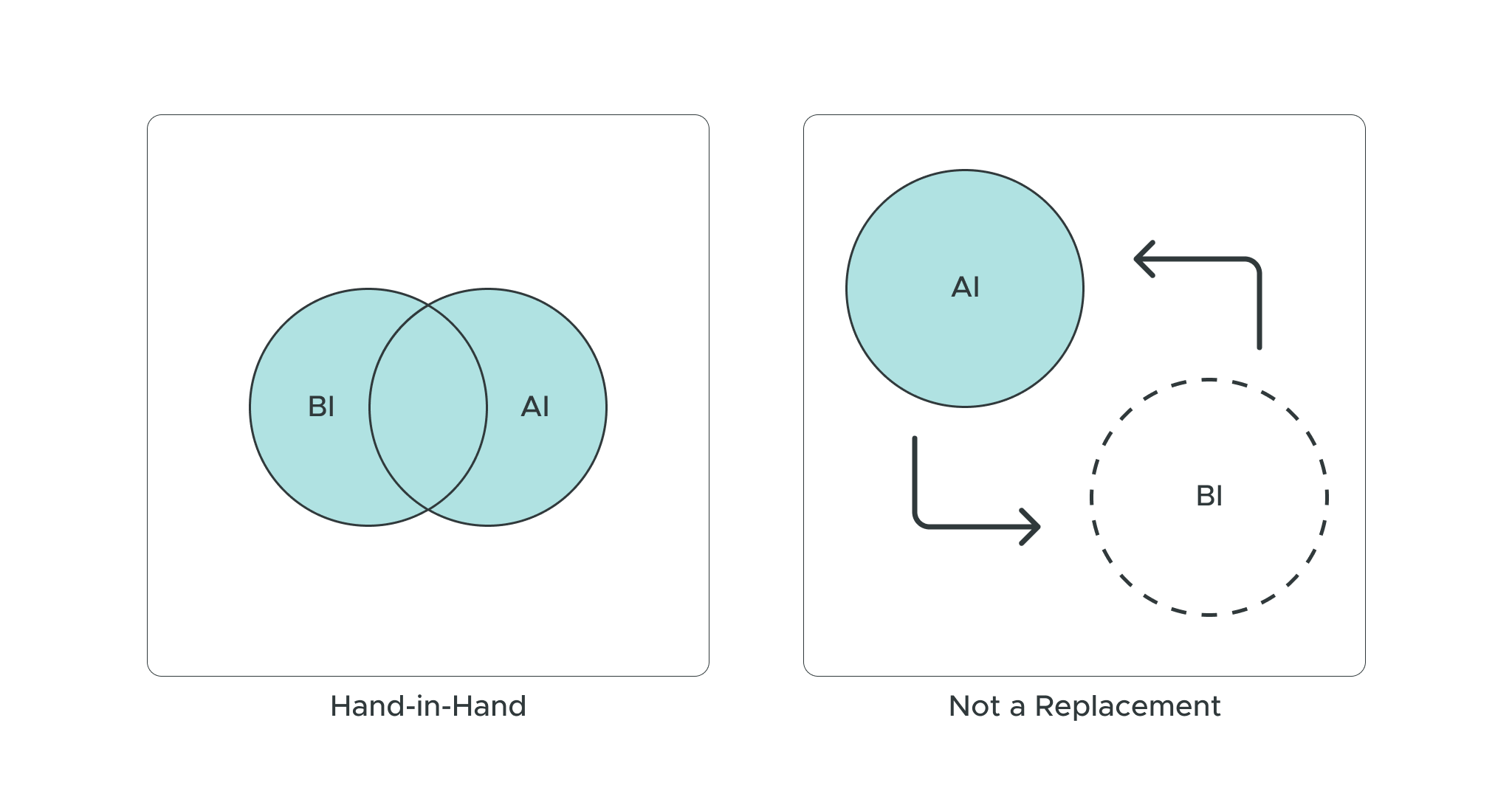
Recently, I was talking to the head of technology at a retail conglomerate. The executive was actively planning his foray into data science and asked me, “How can we make the transition from business intelligence (BI) to artificial intelligence (AI)?” The question made me uneasy.
This is a query I often get from leaders evaluating advanced analytics. But, what worries me are the two key misconceptions bundled in this apparently benign question.
- People often assume that AI can replace BI.
- Many people assume capabilities in AI are a sign of higher maturity.
Both these assumptions are seriously flawed.
Let’s bust these myths and find out why one needs to align BI and AI to deliver business value. We’ll examine the real drivers of decision maturity and understand what it takes to scale data-driven decisions in an organization.
Why Upgrading from BI to AI is a Misnomer
Before talking data or analytics, let’s take a step back and ask what is the purpose of data analytics.
As we discussed in a previous blog post, the goal is to deliver actionable insights that drive effective, impactful, and timely decisions. The discipline that enables this across an organization at scale is called Decision Intelligence (DI).
To make a business decision, users often need answers to many types of questions. Let’s take the example of a sales head at a retail firm. To meet the organization’s annual goal of 35% revenue growth, the sales lead needs to continuously answer the following kinds of questions:
- What was our customer base last month, and which geographies grew?
- Why did North America lose market share two weeks ago?
- How many customers can we expect to acquire next month through our online channel?
Each of these questions need a certain type of analytical insight. The first calls for descriptive analytics – simple summaries of backward-focused insights. The second needs diagnostic analytics – answers provided by causal analysis. And the third requires predictive analytics – deeper insights that give us a glimpse into the future.
The answer to descriptive (‘what’) questions are often delivered by BI. Whereas, the diagnostic and predictive (‘why’ and ‘how’) questions are usually answered through statistics and machine learning, often clubbed under AI.
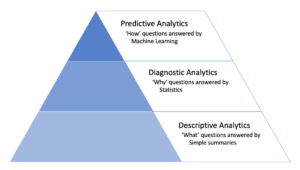
Kinds of business questions and the types of analytics insights
Going by the above example, it’s obvious that every business needs answers to both simple and deeper questions. Hence, it’s critical that BI and AI are aligned, working hand-in-hand to enable outcomes. After all, you don’t stop walking once you learn to run.
(In case you’re wondering why prescriptive analytics, or recommendations for business action, is missing in the above list, it is not a separate type of analytics. Every type of insight, whether descriptive, diagnostic, or predictive, must always be supplemented with a prescriptive recommendation. Or else, what’s the point?)
Why AI is Not Necessarily a Sign of Superior Decision Maturity
There’s one challenge with the pyramid visual included above. People mistake AI’s higher position to be a sign of maturity. This is the second fatal assumption in the executive’s question.
If a company is able to do projects with AI, has it leveled up? Not really.
In the second blog in this series, we explained the three disciplines of decision intelligence:
- Cognitive science, to better understand the decision-maker and their audience
- Data analytics, to answer business questions with data insights
- Organizational intelligence, to contextualize decisions and influence outcomes
Clearly, analytics is just one of the ingredients of data-driven decisions within an organization.
One must first understand the decision-makers’ goals, priorities, and pain points. This calls for domain and solutioning skills (in people) to translate the business needs into a form that can be solved with data and analytics. Once insights are identified through data analytics (technology), they must be converted into actionable recommendations. This complete decisioning cycle should be built into the business workflow (as a process) to make it frictionless and repeatable for users.
I’ve come across organizations that have not ventured beyond BI, but their ability to decision using descriptive insights is very robust. On the other hand, there are firms with AI models in production that stumble to answer business questions and drive organizational decisions.
A company’s capability in predictive analytics is no guarantee of its maturity in data-driven decisions.
We’ve seen why organizations need to align BI and AI, and how their combined abilities across people, technology, and process are crucial for decision maturity. But, how does an organization get there?
Leaders must work on creating and fostering a data culture, embracing modern data platforms, and setting up a scalable operating model to promote data-driven decisions. In the next section we’ll present a sound approach to build modern data platforms.
Treat Data like a Product to Scale Decision Intelligence
As organizations build BI and AI capabilities to answer business questions, the technology landscape increases in complexity. What drives this? Traditionally, every analytics solution is built by identifying data sources and curating data feeds needed for each solution.
For example, when teams address the three BI and AI use cases described in the earlier section, they typically build three different data pipelines and data stores. This duplicates the data engineering and transformation efforts. As teams build multiple solutions, the data architectures are difficult to maintain and often, impossible to scale.
Alternately, it is empowering to treat data like a product. What’s the fundamental difference? Instead of working backward from the end business use cases and creating siloed upstream systems for each one, this novel approach designs data stores, each of which can then serve multiple business use cases downstream.
How can data products bring to life the three use cases discussed earlier? Teams would first design a Customer Data Product that provides a 360-degree view of customers. This is achieved by tapping into all transaction and master data sources, such as demographics, purchase transactions, customer service, feedback, and social channels.
Once a Customer Data Product is built, each of the three use cases across BI and AI can be served by it. As for data lakes and data warehouses — data products are designed to sit on top of these traditional data platforms. This approach helps move away from monolithic, one-size-fits-all warehouses or lakes to distributed data stores that address specific domain questions.
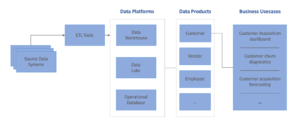
The Data Product Approach (Reference: HBR – A better way to put your data to work)
Data Products thus act as a bridge between the data engineering layer and the data consumption layer — an efficient one at that. They save teams the time spent searching for data, transforming it into the right format, and building data pipelines to feed the BI or AI solutions.
An efficient way to bring a data product to life is by building a common semantic layer platform that supports data model sharing, collaboration, and ownership. A semantic layer enables centralization of data governance and control while enabling de-centralized consumption through data products.
Teams can design and build new data products, for example, for vendors, employees, and services, by leveraging pre-built building blocks from the semantic layer. This approach saves time and effort while accelerating time to market for new analytics solutions, whether it’s a BI or an AI use case.
This is how one architects a modern data platform to scale decision intelligence. When leaders combine this with effective operating models and practices to foster a collaborative data culture, data-driven organizations become a reality.
Note: This is the third post in the blog series on Decision Intelligence. Check out the earlier posts: Why Decision Intelligence is the Most Important Data Analytics Trend of this Decade and How to Implement Decision Intelligence in Your Enterprise With a Semantic Layer.
ANALYST REPORT