February 2, 2023
What Is an AI Center Of Excellence?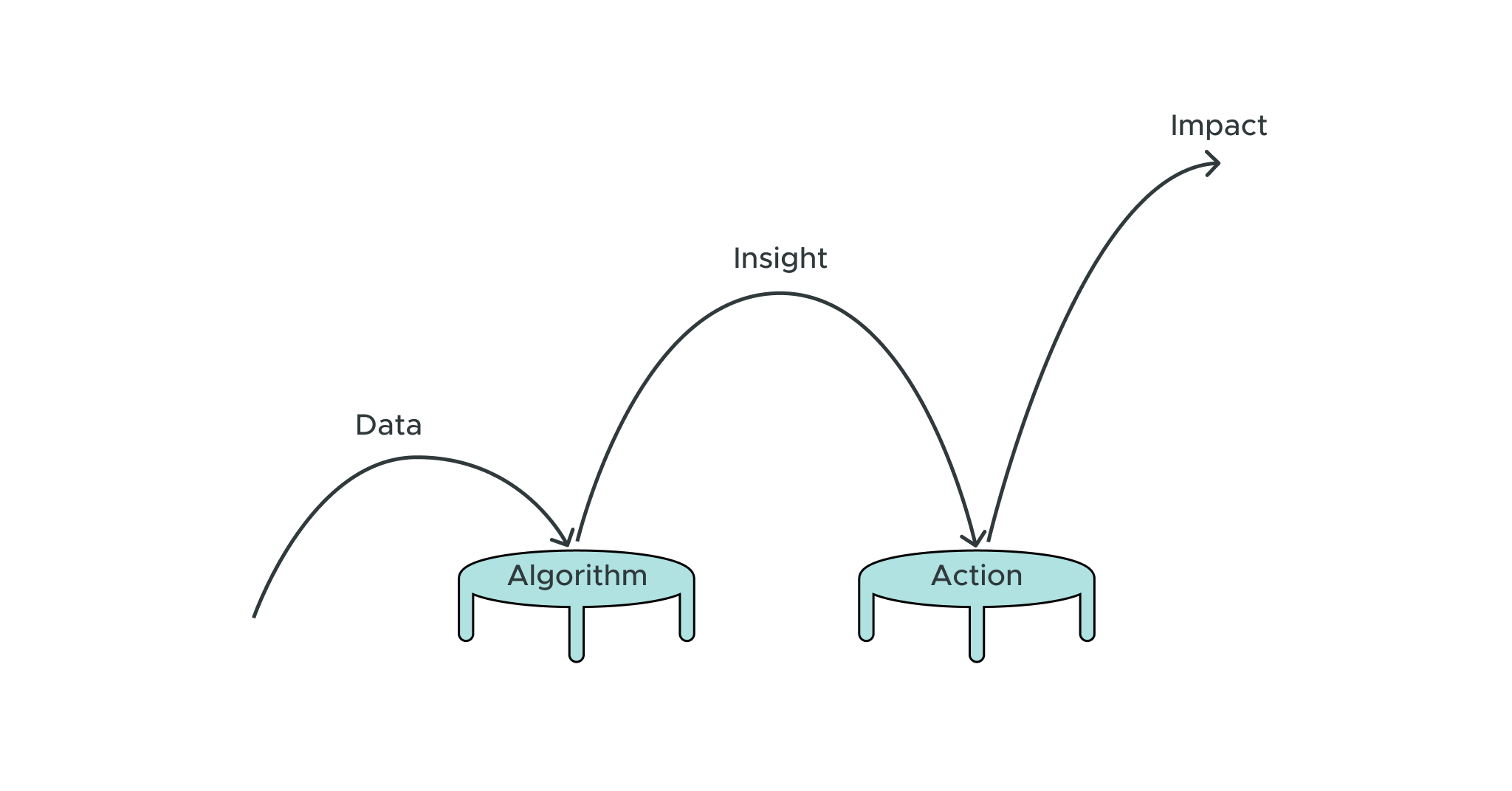
Financial services companies have unique security, regulatory, and compliance obligations. At the same time, the industry is facing rapid evolution and a new generation of FinTech competitors are emerging that aren’t burdened by legacy systems or thinking.
At the center of evolving and remaining competitive is creating an organization committed to making customer-centric decisions to enhance the customer experience. That puts data front and center as a foundational component of meeting customer needs and building a better experience.
The right data strategies can help you dramatically accelerate growth in banking and financial services. For example, consumer data can be merged with company performance data to track initiatives and gauge success. At the same time, data can underpin your compliance and risk assessment to prevent fraud and manage financial risk.
Involving a semantic layer, built on a solid data foundation, can help financial organizations maintain and improve their competitive edge.
How Major Financial Services Leaders Are Deploying Data Strategies in Financial Services
Defining and driving the execution of data management programs requires a top-down approach with accountability and cultural commitment. Data must be treated as a strategic asset and competitive advantage to maximize value. This requires governing and managing the data lifecycle for value creation.
Organizations need:
- A clearly defined data and analytics strategy
- Data democratization to enable access and use
- KPI tracking for employees (balance score cards)
- Identification of business analytics use cases for value creation
- Continuous testing and optimization
Businesses must find the right balance between data monetization and the acceptable use of data. At the same time, data must be a foundational component that informs and influences strategy. In other words, high-performing companies must create an evidence-based culture.
Here are some of the ways major financial service leaders are addressing data strategies to drive their organizations forward.
Aligning AI and BI to Create Better Business Outcomes
You must be disciplined to work with the insights you derive from data to change behaviors that will have an impact on the company, said Juan Gorricho, VP of Global Data and BI Data at Visa. You must have a system for filtering data to align insights with business value for better outcomes.
Everything (and everyone) must be aligned.
You have two key stakeholders in the value chain: data teams and business operations teams. For there to be a business impact, there needs to be a very strong partnership between these two teams. Data teams must have a good grasp of the objective that operations teams are trying to meet. At the same time, alignment requires the ops team to understand how data can help them solve business challenges.
Gorricho says it is important to employ a value framework to optimize AI and BI for business outcomes that must be repeated over and over for maximum value.
Data > Algorithm > Insights > Actions > Impact
Data is the foundational element. You need quality and variety to feed your algorithm. Without quality and variety, AI can create poor models. You need to keep your algorithms simple to maintain focus on the areas that drive business value. In other words, the insights you are looking for must align with operational goals.
While data teams work with the data and algorithms to generate insights, the operations teams focus on the actions, change, and impact. Without alignment, the entire process cannot function effectively.
All of this requires data literacy throughout an organization. There must be an understanding of the role data plays in context and the ability to turn data into use cases and value. This requires consistent discussions between data and operations teams, but everyone must be able to speak data.
Accelerating AI and BI Business Impact with an Effective Data Strategy
Ramdas Narayanan is the VP PM of Data Analytics and Insights Tech at Bank of America. As a hands-on practitioner, he knows that an effective data strategy requires treating data as a product.
Regardless of where your data comes from, it’s important to define the success measures that will tell you whether your data strategy is working, and which consumers or stakeholders that the data will impact.
Treating data as a product means ensuring you have the right data pipelines for your needs. You need to think about data assets as products and your organization’s data scientists, BI analysts, and operations teams as customers.
How can you make sure you have the right data to deliver the insights your customers need to drive value?
Answering that question will frame the design of your data architecture and impact every aspect of your data strategy, including:
- Data pipeline sourcing and transformation
- Data lineage
- Data observability
- BI and visualization
- AI/ML capabilities
- Data governance and management
- Data classifications
Data as a product is a key construct for BI and AI data capabilities. Narayanan says if you want to be successful using AI and BI, you must have data at the center of your foundation for effective insights and predictions.
He thinks of the process as an endless loop that includes data lakes and warehouses, AI and ML, and BI and Reporting. Data no longer flows from A to B, instead, it flows continuously which means it also needs to be frequently evaluated as new data is gathered. At the same time, it requires a continuous refinement of the metrics based on data drift and model evaluation results.
The key drivers for artificial intelligence and business intelligence cross over from data governance, cloud architecture, and your data science platform. This includes:
- Your data mesh architecture
- Collaboration between IT and business teams
- Rapid prototyping and experimentation
- Organization for scalability
Creating and Fostering a Data-Driven Culture
Driving an analytics transformation requires more than just the technical capabilities, according to Jon Francis, Chief Data & Analytics Officer at PayPal. While you must have the right data sources, technology, and skills to produce the insights you need, you also need to create a data-driven culture within your organization to maximize value.
Lead with Empathy
Francis says to build this culture, you have to lead with culture. Whether you’re working with a marketing or operations team, for example, you need to understand what they are trying to accomplish, what their challenges are, and the data they need to move forward. Without his knowledge, it becomes difficult to leverage analytics to help provide the insights they need for better outcomes.
Often what gets overlooked in analytics is the role of change management. It’s not enough to identify solutions, but you also need to be able to bring along the stakeholders you are working with.
Think like a Business Owner
You must also think like a business owner to identify the KPIs that drive an organization and then apply analytics solutions that can help improve upon those KPIs.
Avoid Shiny Objects
There is often a tendency in data science to build new solutions. There’s technology available today that didn’t exist a few short years ago and it’s exciting to be on the cutting edge. However, if you aren’t focused on the areas that drive value, it’s easy to get distracted by shiny objects. You cannot afford to build solutions looking for products. You must maintain a product focus first and create data models to address the key business drivers.
Pathway to Scale
It’s also important that when you think about proof of concept, you always think about scale. For a solution to be effective at enterprises, you have to be able to scale to leverage data strategies for maximum impact.
Set Expectations for Testing and Learning
Consumer behavior and competitive business environments mean things are constantly evolving and changing. You need to build an environment and culture that understands there is a continuous evolution taking place and that you must nurture the data and tech lifecycle.
Building a test and learn cycle that evolves with the data and operations goals is essential to deriving value. At the same time, you also have to manage evolution. It can be too easy to continue to build new products, which then requires more management and support — which can then limit your bandwidth for new projects.
Making Advanced Analytics and Insights More Actionable
Culture is how we get things done, said Ratan Tadiparthi, VP, Head of Data & Analytics at Fifth Third Bank.
You can generate all sorts of data and insights, but if you are unable or unwilling to act on them, you won’t move your organization forward. According to NewVantage Partners 2022 Data and AI Leadership Executive Survey, the principal challenge to becoming a data-driven organization is not the technology, but the people – and that’s only accelerating. In the 2018 version of the survey, 19.1% of executives chose technology limitations and 80.9% chose people and processes as their greatest challenges. In 2022, only 8.1% chose tech limitations while 91.9% said people and processes.
It’s not just the data or strategy that leads to change. In reality, it’s the underlying culture that drives the execution of the strategy that creates value. Business consultant Peter Drucker famously put it this way: “Culture eats strategy for breakfast.”
Tadiparthi envisions the value chain as a pyramid. At the wide base is trusted data which forms the foundation. At the next level are transformation initiatives and KPIs. Business metrics site on top of that from which you can derive business value. At the very top of the pyramid is where you find the actionable insights.
Each strategic step must build on the one beneath it to lead to insights that can be turned into action. However, you must understand the “why” at every step. What questions are you trying to answer, to influence which outcomes, to solve which problems? Understanding the answers will help you execute projects more efficiently by helping you manage priorities, resource allocation, and collaboration to deliver outcomes.
Making advanced analytics and insights more actionable requires a high level of data literacy throughout an organization. Data must also be trusted and frictionless. Users must have an easy way to access data and understand its implications.
Data Strategies in Financial Services
Financial companies have been obsessed with improving the customer experience for years. Achieving these goals requires analytics around customer behavior. This helps companies anticipate customer needs and predict where the marketplace is going.
Focusing on data science, producing actionable insights, and delivering impactful change requires alignment among everyone involved in the data chain. It requires an effective data strategy that treats data as a product to support stakeholders and a data-driven culture that allows organizations to maximize value.
When organizations have high data literacy and an understanding of change management, data can unlock the insights needed to accelerate growth.
RESEARCH REPORT