October 18, 2019
Should You Build or Buy Your Universal Semantic Layer?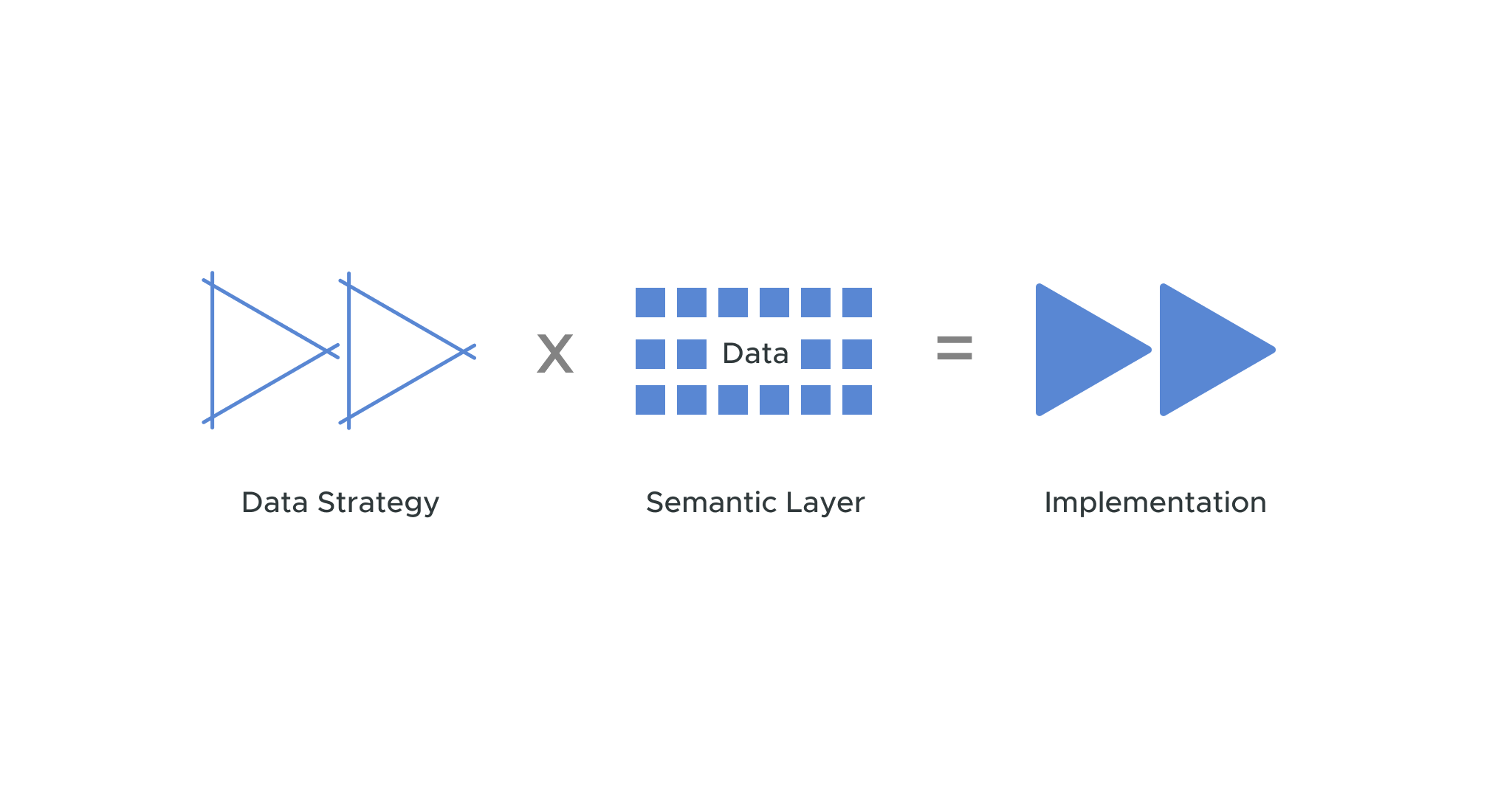
This blog is part of a series from Vin Vashishta, Founder and Technical Advisor with V Squared. V Squared advises businesses on AI strategy, data product strategy, transformation, and data organizational build-out services. They have helped clients deliver products with $100M+ in ARR and built small (5 data professionals) to large (30 professionals) D&A organizations. V Squared clients include Airbus, Siemens, Walmart, JPMC, as well as a wide range of smaller to medium businesses. Follow Vin on LinkedIn, Twitter, YouTube, and his website.
—
Semantic Layers and Data Strategy
The semantic layer is at the heart of a significant challenge facing businesses that need to monetize their data. The data maturity journey begins with data in multiple repositories controlled by different units. Data lives where it is gathered and consumed, solutions are often close to business needs.
The implementation sounds good, but it isn’t scalable. And today’s businesses need to scale data and AI to handle more use cases. Decentralized data is a roadblock to maturity and value generation.
When that connection isn’t clear, the initiative to centralize data is typically driven by the data team. It’s a data best practice, and that’s an excellent justification for the technology team. The semantic layer is communicated as a technical solution to centralize data and implement best practices.
But, data best practices aren’t relevant for the rest of the business. Presenting semantic layers tactically means that they have no connection to value streams or the larger goal of scaling data and AI. Data teams spend multiple meetings explaining technical value, but other teams don’t hear any links to their goals.
On one side, there are business units with a workable solution in place, but don’t see the point of making the change to their centralized solution. On the other side, there are data teams who don’t understand why the rest of the business isn’t bought in.
A theme I see repeatedly is that no one adopts what they don’t understand. For business units to change, there must be significant, obvious value. Data strategy is the bridge between value creation and technical solutions.
Balancing Centralized Data With Decentralized Business Needs
The resistance starts when business units feel like they are losing control over their data. Teams own their data-gathering process to ensure that it meets their specific needs. So if data is centralized, they feel like they’ve lost control.
Teams are reliant on data to complete their jobs. Their operational efficiency and value creation are connected to their data ownership. Giving another team control over mission-critical data does not seem like the best decision.
Other business units gather and leverage data in different ways. Centralization isn’t associated with flexibility. In the software paradigm, solutions are monolithic. They are built to meet narrow requirements vs. generalized to meet a broad set of needs.
But, data is a different asset class because it can be reused across business units and cases. A dataset isn’t a rigid resource like software. Decentralized data isn’t a threat to customized solutions when a semantic layer is implemented.
The next barrier to adopting a centralized data solution is the perception that the data team will become a bottleneck. Business units now have some control over solutions development. Centralization implies they will have to request resources and go through a lengthy process to get solutions built for them. The data team will control the centralized data store, making people see them as gatekeepers.
The semantic layer provides a solution for this challenge as well. Users have greater control over solutions development and rapid deployment when the right tools are implemented.
A semantic layer and self-service tools overcome the challenge of meeting diverse needs while implementing a technical best practice. Technology meets value creation.
What’s The Purpose Of A Data Strategy?
Data strategy informs decision-making across the business. It’s a thesis of value creation that explains why the company uses data to create and deliver customer value. According to Accenture, firms with a holistic data and AI strategy see 50% higher revenue growth than their peers.
Implementing best practices results in higher-quality data. The business saves money because effort isn’t duplicated, and redundant tools aren’t supported. The business generates new revenue because the centralized solution is scalable.
A consistent data governance strategy is possible with centralized data. The business can start treating its data like an asset and monetizing it in a structured way.
Data security also needs centralization to be effective. Data scattered across multiple systems is challenging to control and track access to. Breaches happen in the dark and vulnerabilities go undetected. The business can implement a data security framework alongside their data strategy to reduce costs and risks.
Each business unit has identified opportunities to create and deliver value. Data should support, not replace or disrupt those opportunities. There must be an alignment that’s driven from the top down.
For instance, marketing teams are dealing with reductions in advertising budgets and pressure to increase ad campaign effectiveness. Data is an integral part of that initiative. How does centralizing data support the marketing team?
Data becomes more consistent when there’s a data strategy in place. The data team has experts in data gathering, cleaning, reconciling, and serving. Why does that create value for the marketing team’s campaign initiative? Reporting is more accurate, insights are more reliable, and campaign selection decisions result in more effective advertising.
That’s how a data strategy connects technology to business value. The business objective is higher-quality data to improve how data supports strategic goals across teams. The implementation is a data centralization initiative. But where do the semantic layer and self-service tools fit into the picture?
How The Semantic Layer Supports Business Needs
The semantic layer is a data strategy implementation enabler. Marketing needs access to their data through reports that deliver insights about campaign effectiveness. If the data team becomes a bottleneck, that jeopardizes their success. The semantic layer facilitates access to data and puts solutions development into the hands of users.
Self-service is a value generator for the data team too. The less time they spend on ad hoc requests, the more focused they can be on delivering high-value initiatives. It reduces staffing levels, which is critical in the current talent shortage.
This puts self-service tools into the strategy enabler category. It also shows a value proposition for data literacy training. The tools are only one part of the value creation puzzle. People at the front line must upskill to use those tools.
This is the implementation justification. Why does marketing need a semantic layer? Based on the data strategy, the team understands their need for access and self-service tools. The semantic layer is the technical solution that best meets their business needs.
What else does the marketing team need? New tools that provide access to the semantic layer and help them develop solutions. Those will help them deliver data products faster and leverage their domain expertise to create solutions that meet team needs.
Data literacy and training are also required. New knowledge and capabilities will allow them to create self-service tools. They will retain control over the solutions required to succeed in campaign optimization.
Demystify Data Products To Increase Adoption
Combining data strategy with implementation tools like a semantic layer and self-service solutions makes the connection to value apparent. Data and AI feel like a black box to users until they understand the benefits to their role.
Demystifying solutions starts by taking the technology out of the conversation. That’s why terms like semantic layer are helpful. All of the technical jargon and implementation details are on the other side. The semantic layer handles business needs around access to centralized data.
When someone asks, “What’s a semantic layer?” Users can give a simple response: “It’s how we access our data to make better ad campaign selection decisions.”
“What’s a self-service solution?”
“It’s the software we use to build reports about campaign performance.”
Teams buy in when the connection between technology and their roles becomes obvious. The value of pushing data strategy across the enterprise goes beyond alignment. Data and AI are easier to understand for front-line workers. They’ve regained control over their workflow, data, and solutions.
With data literacy training and high strategy awareness, people across the business use data more effectively. Data scales to handle more use cases, and the technology achieves its potential for high value.
ANALYST REPORT